Publikationen
Does Regularity Captured in an LMS Relate to Students' Performance in Courses of an Online Degree Program? A Case Study
February 2025
In this paper, we consider an online study program that is attended by students who mostly work full-time and therefore study part-time. We investigate whether relationships can be found between students’ interactions in online courses of this program and their performance in courses. Though the study program and the courses are both online, their delivery through the learning management system (LMS) Moodle has not been designed to store comprehensive student interactions automatically. Therefore, we investigated whether the weekly regularity of students in the LMS is correlated to their performance in the course. Weekly regularity has been introduced in the context of MOOCs (Massive Open Online Courses) in [5]. We obtained inconclusive results. In only a few courses we could find a strong or moderate correlation. We conjecture that this might be due to two factors: the limitation of our dataset and the fact that, in the context of an online degree program, successful students do not necessarily access the learning material on a regular weekly basis.
Dogaru, T., Merceron, A., Fröhlich, J., & Sauer, P. (2025). Does Regularity Captured in an LMS Relate to Students‘ Performance in Courses of an Online Degree Program? A Case Study. Zenodo Preprint. https://doi.org/10.5281/zenodo.14900710
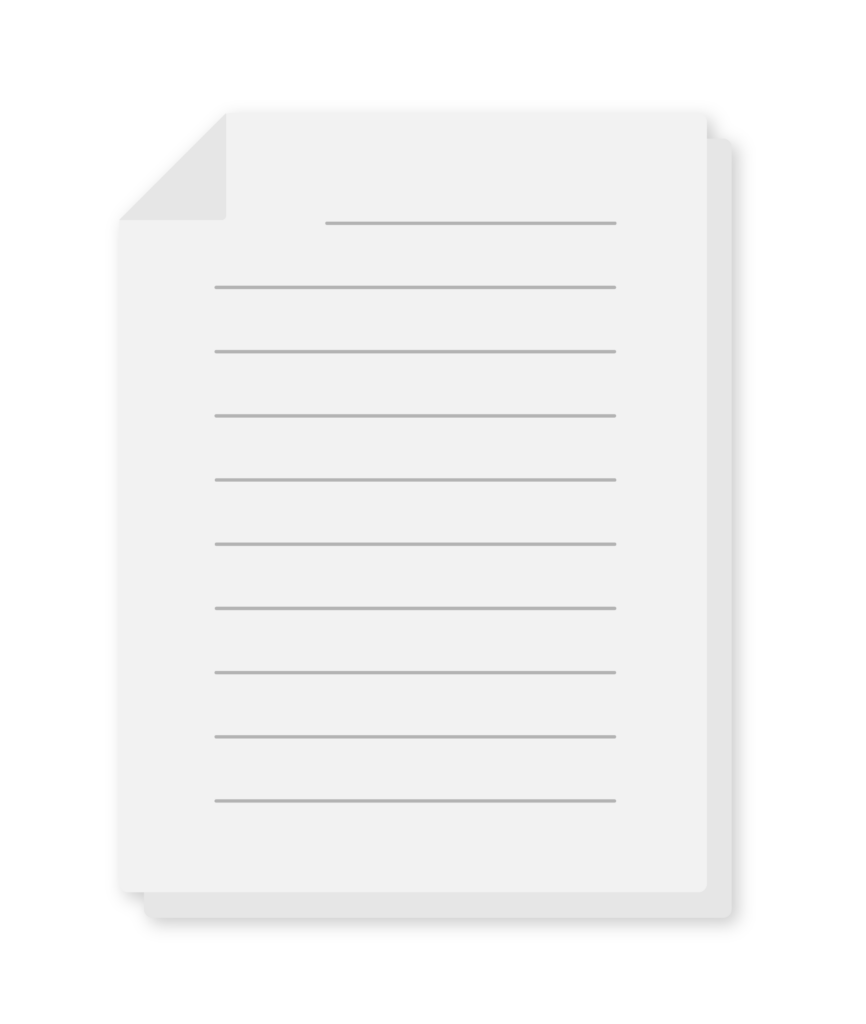
Open Educational Datasets from the DiSEA Project
November 2024
The Open Educational Datasets from the DiSEA Project contain four datasets. Two datasets contain the aggregated academic data of students from two different degree programs: the Bachelor of Media Informatics (data from two locations) and the Bachelor of Business Informatics (data from one location). Another data set contains the aggregated Moodle log data, which is broken down per course and student. The fourth data set links Moodle log data and the grades in the course.
Dogaru T., Fröhlich, J., Waghela, R., Merceron, A., Sauer, P. (2024). Open Educational Datasets from the DiSEA Project. https://zenodo.org/records/14025857
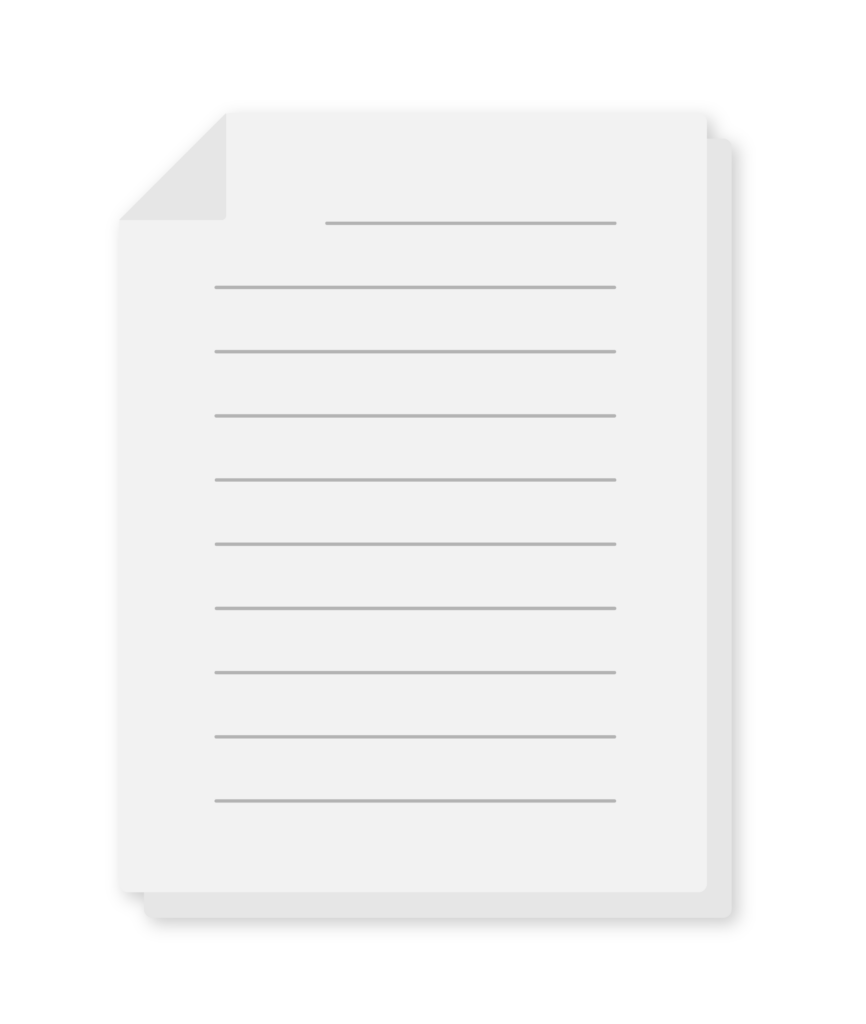
Predicting Dropout in an Online Degree across Two Institutions - A Case Study
September 2024
In this paper, we investigate predicting students at risk of dropping out using academic data from an online study program offered at two different universities. In this program, most students work fulltime and thus study part-time. We use the algorithms decision tree and logistic regression, which have given good results in other works and are interpretable. The decision trees or the coefficients produced by logistic regression can be shared with stakeholders to identify courses that impact dropout the most and that could be considered for additional support. Our results are comparable to the results that others have obtained when considering face-to-face study programs. We propose a preliminary approach to identify courses that impact the prediction the most. Our results also show that merging the data from the two universities is not helpful for the prediction. This might be due to differences in the regulations that impact how students study.
Merceron, A., Dogaru T., Waghela, R., Harper, T.S. Sauer, P.: Predicting Dropout in an Online Degree across Two Institutions – A Case Study. In Proceedings of the Machine Learning and Principles and Practice of Knowledge Discovery in Databases – International Workshops of ECML PKDD 2024, Vilnius, Lithuania, September 9-13, 2024. To appear December 2024.
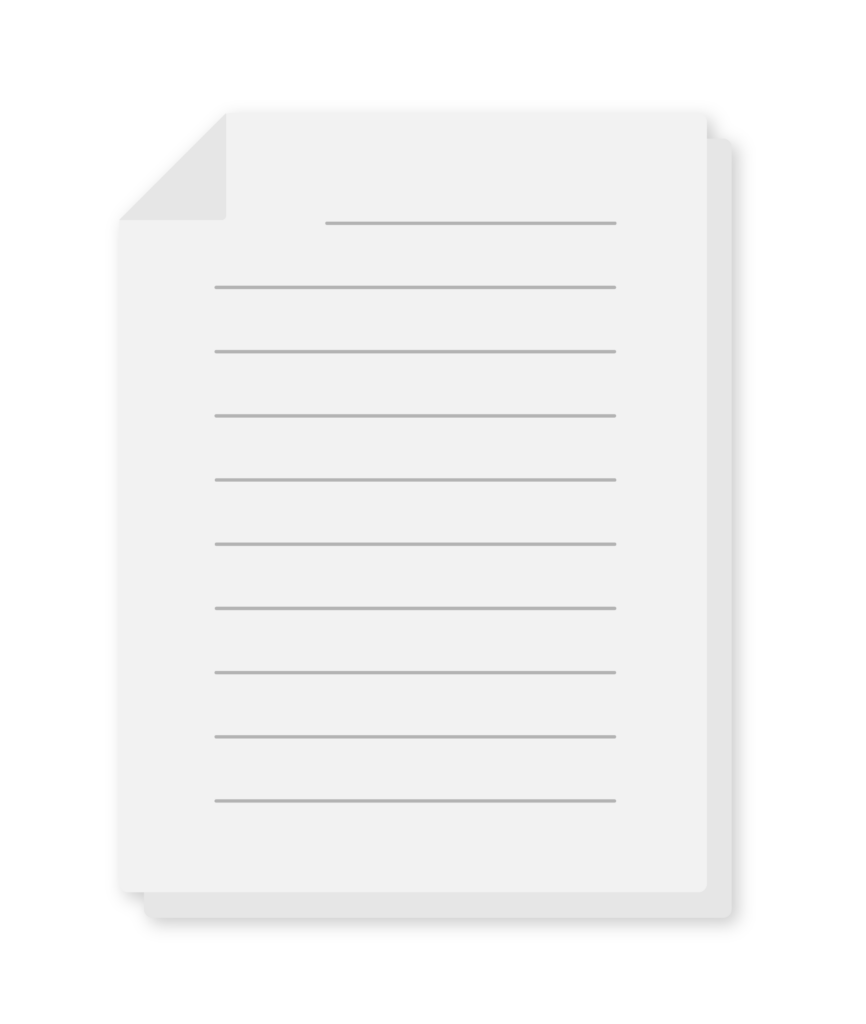
From Low Fidelity to High Fidelity Prototypes: How to Integrate Two Eye Tracking Studies into an Iterative Design Process for a Better User Experience
July 2024
The aim of this study is to investigate the effectiveness of an iterative evaluation design process using low-fidelity prototypes (LFPs) and high-fidelity prototypes (HFPs) for a learner dashboard (LD) to improve user experience (UX) within an eye-tracking study with thinking aloud. The LD itself is designed to support online students in their learning process and self-regulation. Two studies were conducted, Study 1 focused on an LFP and Study 2 on the HFP version of the prototype. The involved participants (n=22) from different semesters provided different perspectives and emphasized the importance of considering heterogeneous user groups in the evaluations. Key findings included fewer adjustments required for the HFP, highlighting the value of early evaluation and iterative design processes in optimizing UX. This iterative approach allowed for continuous improvement based on real-time feedback, resulting in an optimized final prototype that better met functional and cognitive requi rements. Comparison of key concepts across both studies revealed positive effects of methodological improvements, demonstrating the effectiveness of combining early evaluations with refined approaches for improved UX design in learning environments.
Drzyzga, G. and Harder, T. (2024). From Low Fidelity to High Fidelity Prototypes: How to Integrate Two Eye Tracking Studies into an Iterative Design Process for a Better User Experience. In Proceedings of the 19th International Conference on Software Technologies – ICSOFT; ISBN 978-989-758-706-1; ISSN 2184-2833, SciTePress, pages 485-491. DOI: 10.5220/0012854900003753
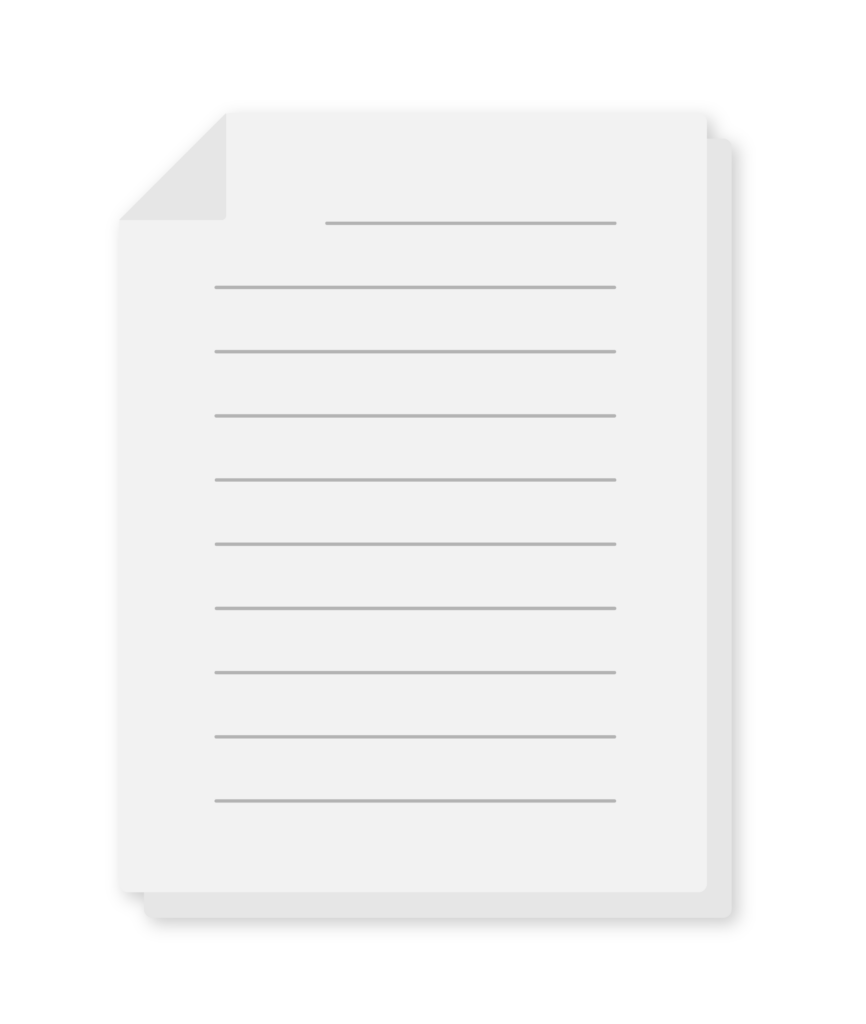
User-Centered Design and Iterative Refinement: Promoting Student Learning with an Interactive Dashboard
November 2023
The study uses a user-centered design methodology to develop a prototype for an interactive student dashboard that focuses on user needs. This includes iterative testing and integration of user feedback to develop a usable interface that presents academic data in a more understandable and intuitive manner. Key features of the dashboard include academic progress tracking and personalized recommendations based on machine learning. The primary target audience is online students who may study in isolation and have less physical contact with their peers. The learner dashboard (LD) will be developed as a plug-in to the university’s learning management system. The study presents the results of a workshop with students experienced in human-computer interaction. They evaluated a prototype of the LD using established interaction principles. The research provides critical insights for future advancements in educational technology and drives the creation of more interactive, personalized, and easy-to-use tools in the academic landscape.
Drzyzga, G. and Harder, T. (2023). User-Centered Design and Iterative Refinement: Promoting Student Learning with an Interactive Dashboard. In Proceedings of the 19th International Conference on Web Information Systems and Technologies, ISBN 978-989-758-672-9, ISSN 2184-3252, Seiten 340-346.
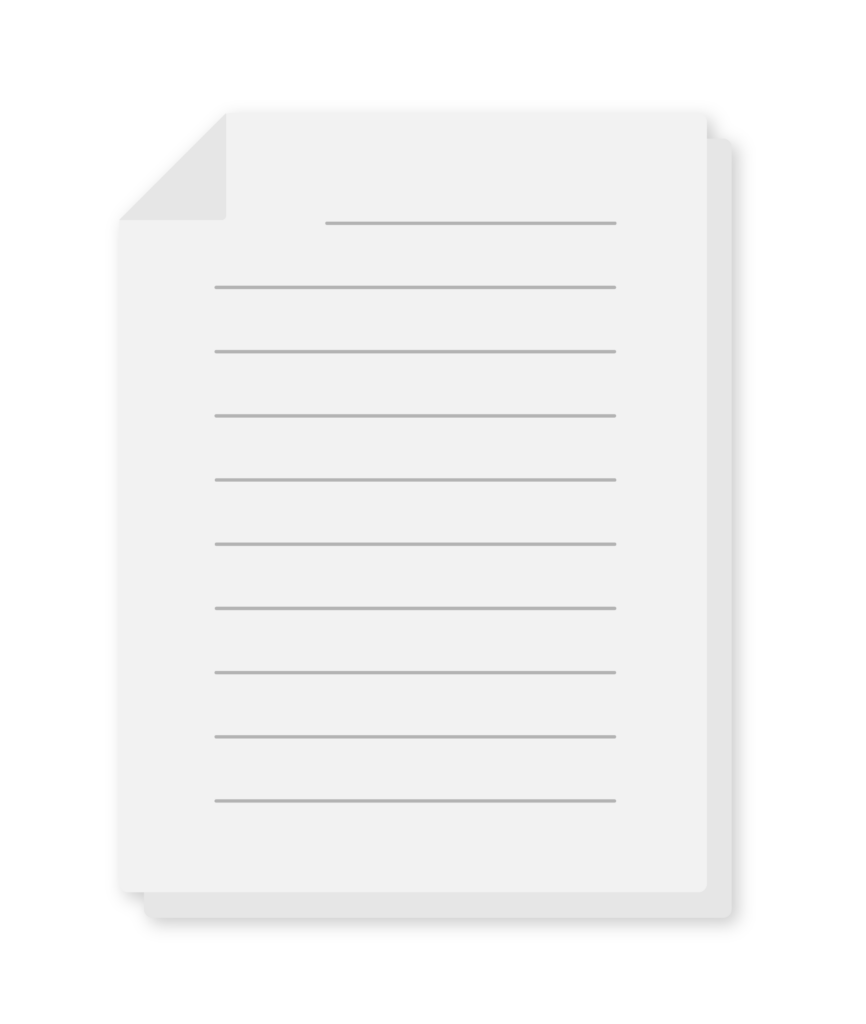
A Three Level Design Study Approach to Develop a Student-Centered Learner Dashboard
November 2023
Online programs risk higher student dropout rates. Supporting learning tools such as learning analytics dashboards (LADs) can promote self-regulated learning and positively impact student outcomes. In this paper, a three-level design study is presented that demonstrates the reduction of cognitive load at multiple levels when students are involved in the LAD design process. Through a user-centered design process (including requirements analysis and expert interviews), a wireframe was developed using participatory methods and evaluated by 24 university students using the laws of Gestalt psychology, resulting in a clickable, low-fidelity prototype (LFD). This was then evaluated by 24 university students using the interaction principles of EN ISO 9241-110:2020. The refined LFD was further evaluated with university students in an eye-tracking study using the thinking-aloud technique (n=10). The feedback emphasized the importance of participatory design and provided critical insights into the most effective use of the LAD and its elements, taking into account cognitive aspects. The results showed significant optimization in the small details and the big picture in the use of content elements, e.g., it is a crucial part to create a navigation structure adapted to the needs of an LAD and it is beneficial to present a reduced level of information during the initial access, with the option to add or access additional elements as needed
Drzyzga, G. und Harder, T. (2023). A Three Level Design Study Approach to Develop a Student-Centered Learner Dashboard. In Proceedings of the 7th International Conference on Computer-Human Interaction Research and Applications, Seiten 262-281.
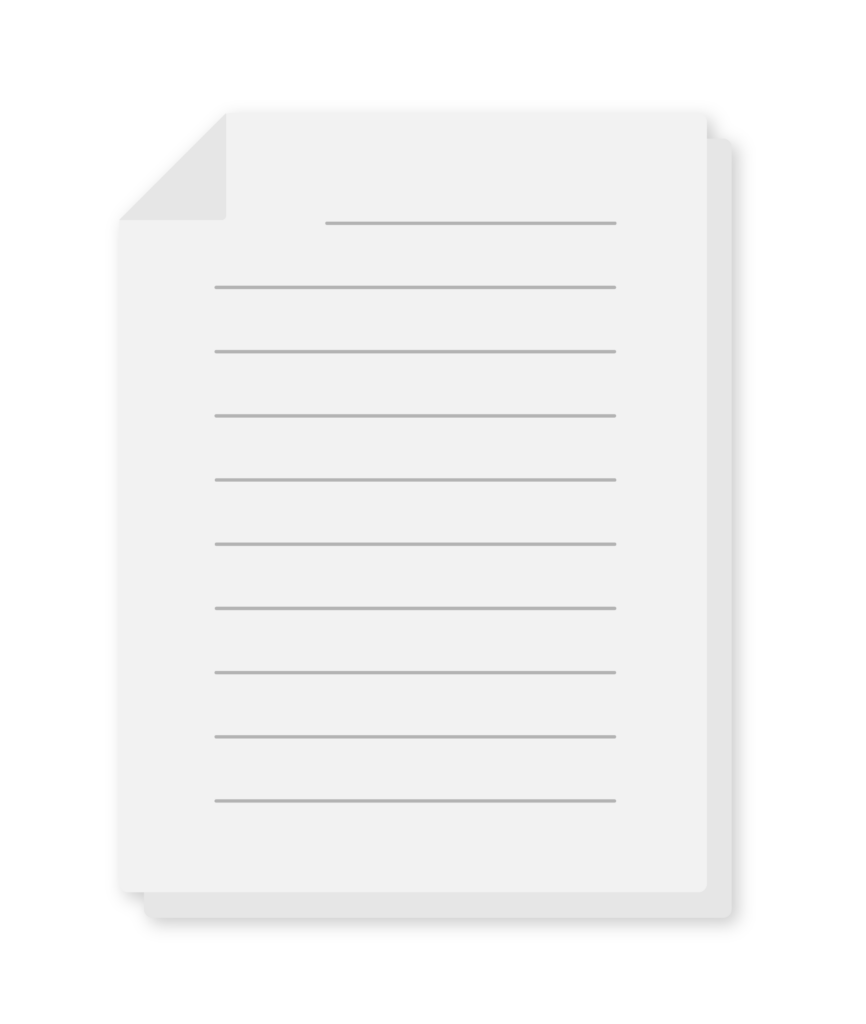
Participative Development of a Learning Dashboard for Online Students using Traditional Design Concepts
November 2023
In order to improve online learning outcomes, a Learning Dashboard (LD) for online students is being developed as a plugin for the learning management system Moodle to support self-regulation. The project itself focuses on the factors that lead to success and failure in online learning. Using a user-centered design approach, the LD will provide students with feedback and functional elements through different cards. 24 online students completed a three-part term paper in which they examined the elements of two wireframes of the LD in relation to Wertheimer’s Gestalt Laws and in terms of factual and interaction problems. We also received 11 card designs from them as a voluntary bonus assignment. Assignments 1 & 2 had to be completed successfully in order to be admitted to the exam. The study was designed to encourage student participation and improve accessibility by taking into account their expertise. The results showed that clearer overviews, clarification of how content elements fit together, more compact solutions, and intuitive controls improved clarity and usability.
Drzyzga, G., Harder, T. und Janneck, M. (2023). Participative Development of a Learning Dashboard for Online Students Using Traditional Design Concepts. In Proceedings of the 7th International Conference on Computer-Human Interaction Research and Applications, Seiten 176-191.
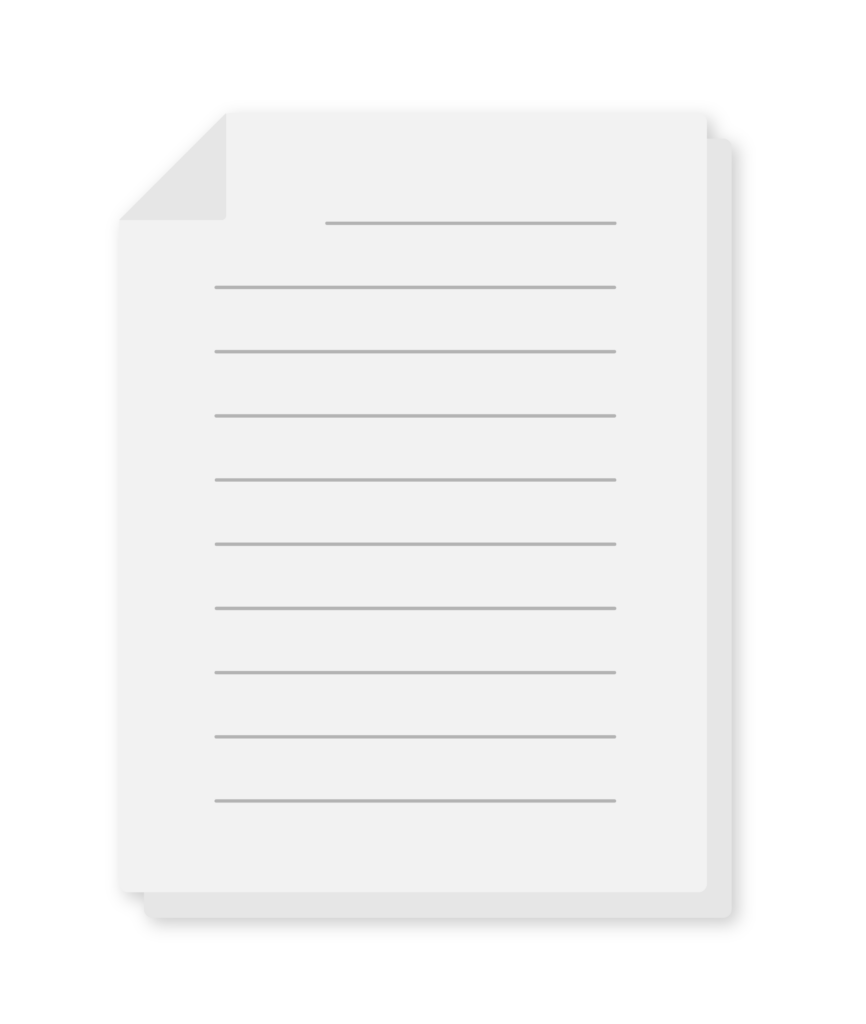
Task Definition in Big Sets of Heterogeneously Structured Moodle LMS Courses
September 2023
Analysing Learning Management System (LMS) log data gives insight into student learning behaviour that can help to predict performance, and as a consequence to avoid drop-out. This contribution provides an application and an adaptation of Rotelli and Monreale’s methodology [RM22] for defining tasks in a set of 10,532 online courses collected from seven universities. Unlike [RM22], we access the log data directly from the Moodle database. Even though our data set is much bigger and more heterogeneous than the one described in [RM22], we could adapt the data selection and filtering, as well as the components’ redefinition and alignment and employ their methodology to define tasks. This work is a contribution to make log data preprocessing open, replicable and more transparent.
Dogaru, Teodora; Götze, Nora; Rotelli, Daniela; Berendsohn, Yoel; Merceron, Agathe; Sauer, Petra (2023). Task Definition in Big Sets of Heterogeneously Structured Moodle LMS Courses. 21. Fachtagung Bildungstechnologien (DELFI). DOI: 10.18420/delfi2023-71
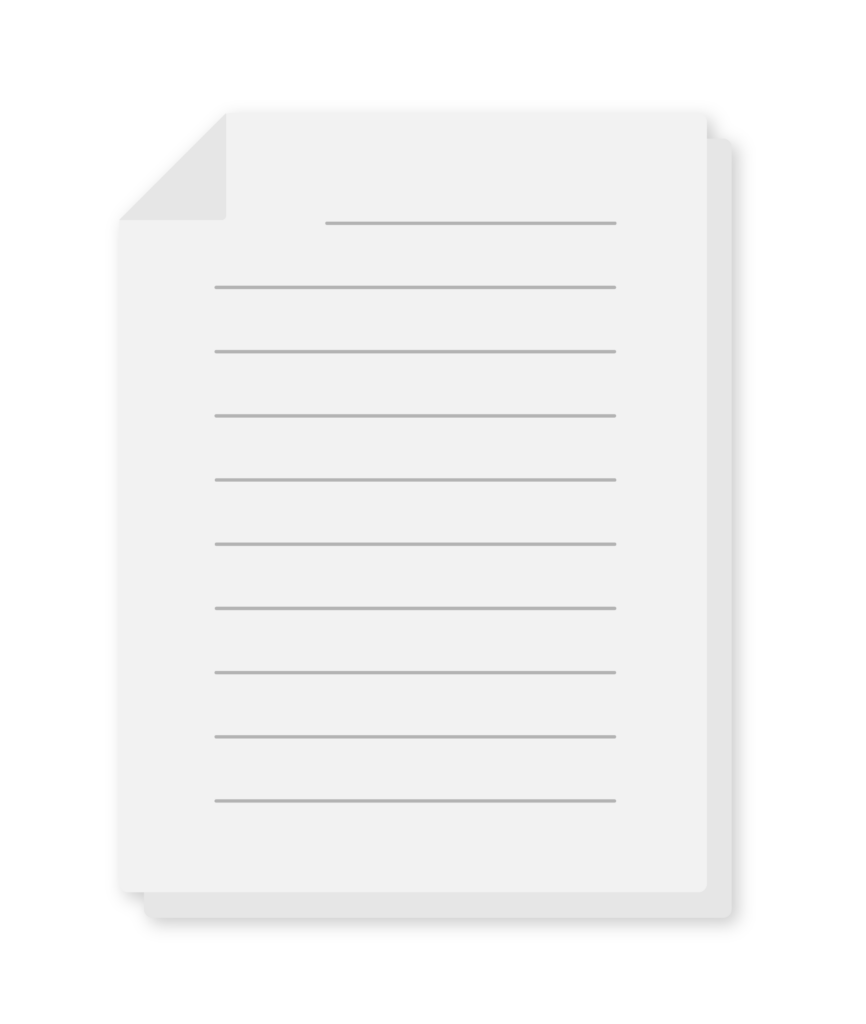
Cognitive Effort in Interaction with Software Systems for Self-regulation - An Eye-Tracking Study
July 2023
The importance of digital degree programs has grown increasingly in recent years, due in part to their ability to provide a personalized learning experience for students. However, degree programs in this format have higher dropout rates than traditional degree programs. In the process of a user-centered design approach, a dashboard for the online degree programs of a university network is developed to provide information and recommendations about the learning process based on descriptive analysis and machine learning (ML) methods. For this purpose, ML models are developed, trained and evaluated. The goal of the dashboard is to promote self-regulation among students and reduce dropout rates. It will be set up as a plug-in through the learning management system (LMS) Moodle exclusively for students. In order to understand which aspects are important for users in relation to the cognitive processes involved in interacting with the dashboard, an eye-tracking study was conducted using the thinking aloud technique. The goal of the study was to investigate which cognitive demands are set for the users when interacting with the prototype and how the automatically generated information is perceived. When integrating the LD into the LMS, care should be taken to ensure that all content is realized in an understandable and easy-to-follow manner, due to the fact that otherwise the effort required to focus on the content elements of the LD could become greater – and with it the cognitive requirements.
Drzyzga, G., Harder, T., Janneck, M. (2023). Cognitive Effort in Interaction with Software Systems for Self-regulation – An Eye-Tracking Study. In: Harris, D., Li, WC. (eds) Engineering Psychology and Cognitive Ergonomics. HCII 2023. Lecture Notes in Computer Science(), vol 14017. Springer, Cham. https://doi.org/10.1007/978-3-031-35392-5_3
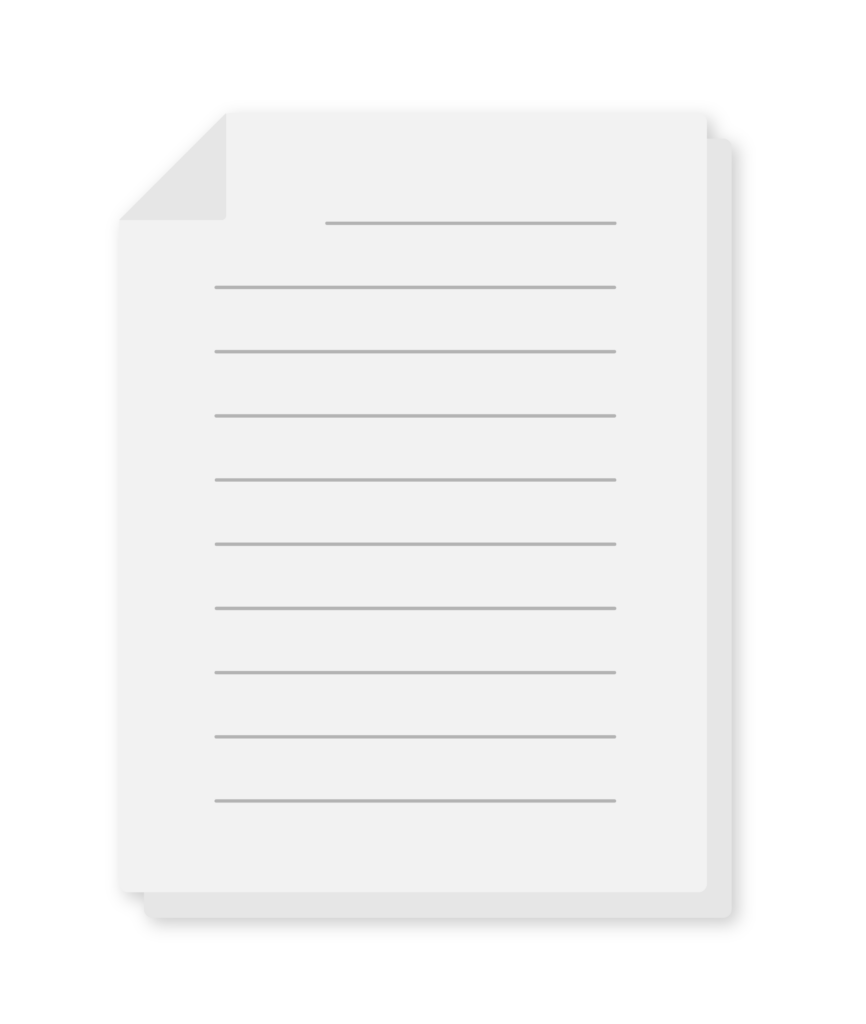
Student-centered Development of an Online Software Tool to Provide Learning Support Feedback: A Design-study Approach
October 2022
Students in online degree programs have a higher risk of dropping out (Diaz, 2002; Beard and Harper, 2002; Baker et al., 2015). The use of learning support tools such as learner dashboards (LD) can promote self-regulated learning, which can have a positive impact on student learning (Jivet et al., 2018; Konert et al., 2016). This paper presents a three-stage design study and shows how the layout of the proposed LD was implemented from the initial digital design to a low-fidelity prototype. First, the developed wireframes were checked for consistency with respect to the Gestalt laws (Wertheimer, 1922). From the resulting wirefame design, a clickable low-fidelity prototype was developed. In the second step, this interactive prototype was reviewed by students (n=24) respect to the seven interaction principles (DIN EN ISO 9241-110, 2020). In the third step, the revised prototype was subjected to an eye-tracking procedure using the Thinking Aloud technique (n=10). The results so far show that the LD should be presented at a reduced information level during initial access, but that this level can be supplemented by additional elements if necessary. The navigation hierarchy should be kept flat and the information should be easy to understand.
Drzyzga, G. and Harder, T. (2022). Student-centered Development of an Online Software Tool to Provide Learning Support Feedback: A Design-study Approach. In Proceedings of the 6th International Conference on Computer-Human Interaction Research and Applications, ISBN 978-989-758-609-5, ISSN 2184-3244, pages 244-248. DOI: 10.5220/0011589100003323
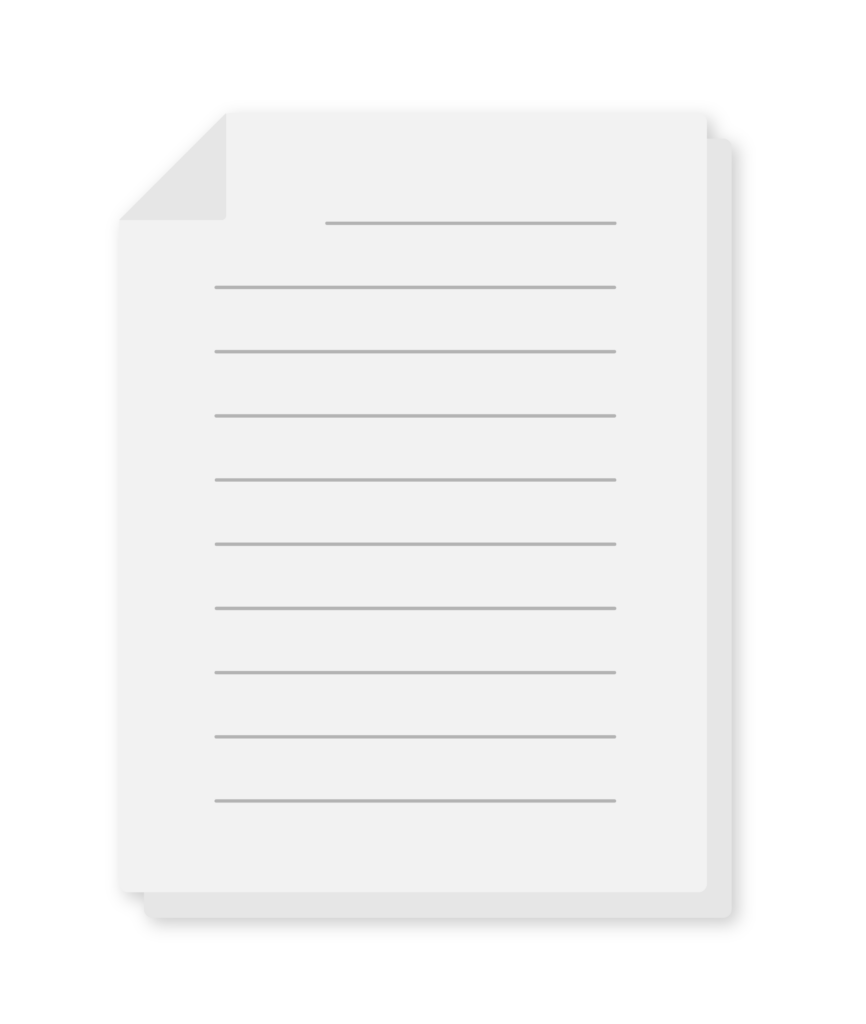
DiSEA: Analysing Success and Dropout in Online-Degrees
April 2021
Although several research works show that students at risk of dropping out of a course or a study program can be predicted with relatively high accuracy, this information has so far often not been accessible to course directors, teachers, or students. The DiSEA project aims to research this issue and close this gap in the context of online-degrees. Building on previous research, machine learning methods will be used to identify risk and success factors. The overall aim is to develop an integrated model to predict success in digital study programs and derive recommendations and interventions for course design, student counseling, and student selfreflection. A user-centered design involving all stakeholders will be followed.
In Companion Proceedings of the 11th Learning Analytics and Knowledge Conference (LAK’21), p. 261-269. Workshop on Addressing Dropout Rates in Higher Education, Online – Everywhere, 2021.
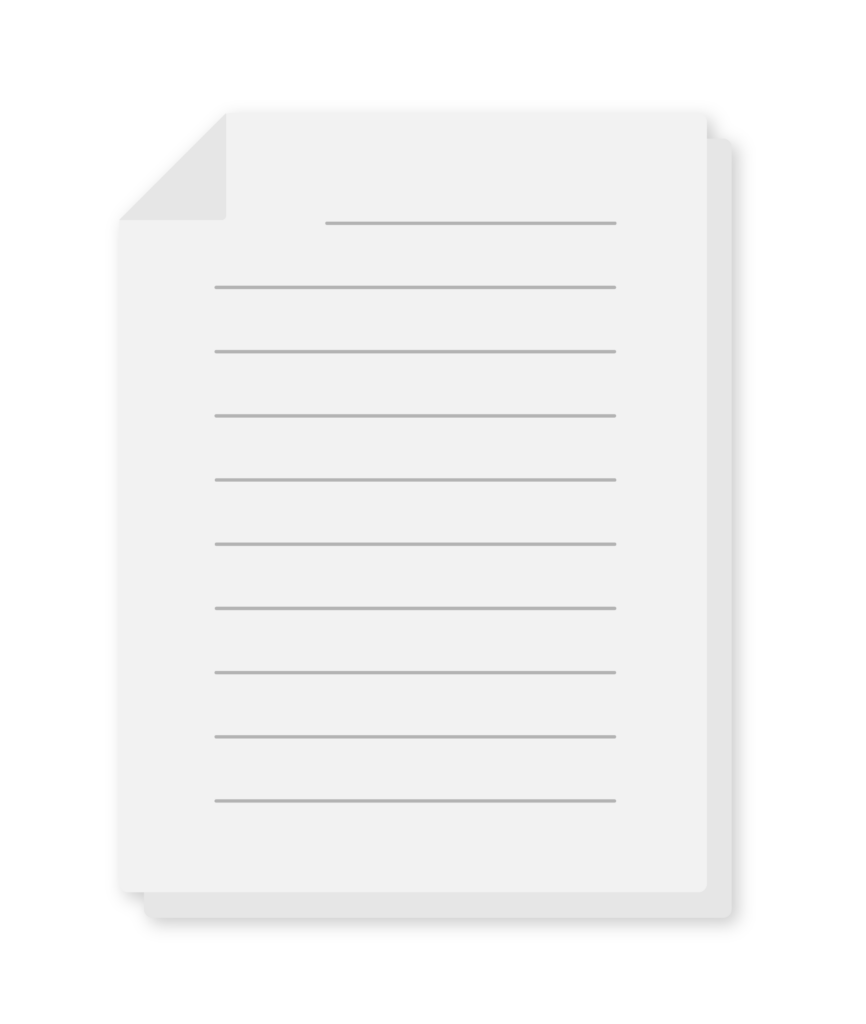